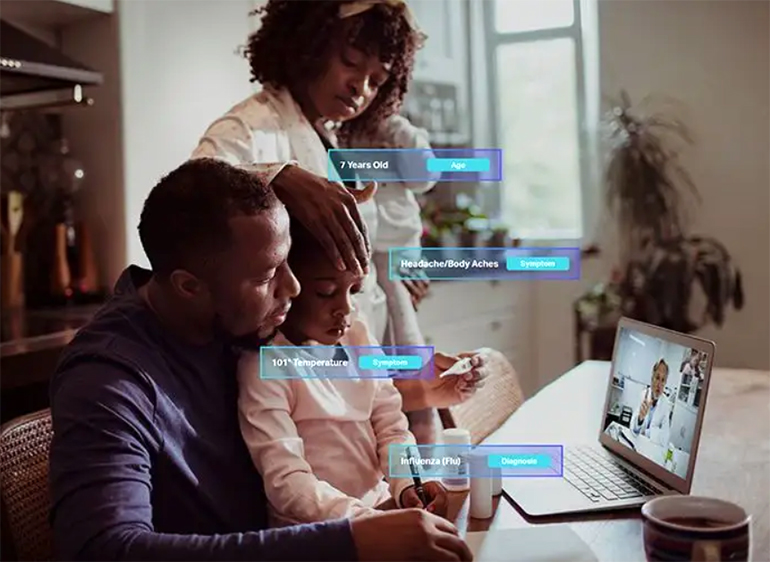
Shaip is an online platform that focuses on healthcare AI data solutions and offers licensed healthcare data designed to help construct AI models. It provides text-based patient medical records and claims data, audio such as physician recordings or patient/doctor conversations, and images and video in the form of X-rays, CT scans, and MRI results.
Like most algorithms, healthcare AI requires diverse data to train machine learning algorithms to identify clinical anomalies, diseases, or cancers more accurately.
Vatsal Ghiya, co-founder and CEO of Shaip, is an expert in improving healthcare AI by using diverse data. Mr. Ghiya recently wrote this article on 6 Ways to Build AI That Incorporates Integrity, Diversity, and Ethics, which provides some additional context for our exclusive interview with him.
Alice Ferng, Medgadget: Tell me about yourself and what interested you and got you started on this “AI journey in healthcare”.
Vatsal Ghiya: I started my journey with a medical transcription company called Mediscribes with my partner, Chetan Parikh, back in 2004. That was our first attempt to get into the medical service industry because in early 2000, offshoring was peaking, and a lot of U.S. physicians wanted to complete their audio transcriptions for the patient at end of the day and wanted to get their work back the next morning to expedite the patient’s records. And with the typical processes that were in place at that time, you know, the in-house transcripts missed a lot and would take a longer time. So that’s how the journey started. In those days, there was no electronic medical recording software. All the dictation was done in a very great length, very great detail, with lots of pages of documentation.
So we did that, and are still doing it. But after six, seven years, we realized that we have all these data coming from different physicians all over the country, as we are primarily focusing on U.S. physicians, and as we were growing all over the country, we have these medical records where doctors are basically kind of, you know, dumping their brain on like, what is happening with the patient? What are the vitals, what are the test results, lab results? What are the follow-ups they are requesting, what medicines they’re prescribing. So that’s where the thought started that what if we can create a technology which can understand these documents, like a physician, and then help physicians make some decisions based on some statistical modeling and things like that. So that’s where forayed into our own company called EZDI (“Easy” Data Intelligence) and started with a journey to understand these medical records. We worked with many large universities, globally, which are very well known in solving this problem. And later we realized that we were embarking on the journey of AI, because AI was not a common word at the time. So that’s how we entered into healthcare AI by creating our own natural language processing technology to understand medical records. And we got the right agreements and licenses in place with the various hospitals and physicians offices to utilize these data to create various products and revenue sharing models. So all those things happened in 2010-11. And then you launch few applications for hospitals, computer assisted coding, computer systems, and CDI (clinical documentation improvement). Basically, the way it works is our technology will create the HL7 interface with the EMR, extract the data, then run our AI algorithms on it understand all the data, medical concepts. Then the first application we had was to help medical coders to optimize the coding. So in a typical inpatient coding environment, medical coders would have to read 10, 20, 30, 40 different medical records to find out group of codes to submit to the insurance companies – our technology would read all the documents and suggest the code. So instead of spending that much time, they can just validate those suggested codes and increase their efficiency with the appropriate coding. You know, reducing the denial and optimizing the coding to get proper reimbursement.
And then now, we were very focused in healthcare. 18 months ago, we were working with Amazon. We are discussing our healthcare, clinical NLP, and how we build our AI and so we can help Amazon to build that area and then we came across the opportunity where they wanted to launch Alexa in 25 different countries. Alexa can understand these local languages outside the USA, so we realized that our company at that time was focused on AI technology and selling those AI technologies to the world and to the hospitals. But there are a lot of companies, large companies like Amazon, and then some other companies who inquire with us who are looking for companies who can help them to build different AI use cases, including and excluding healthcare.
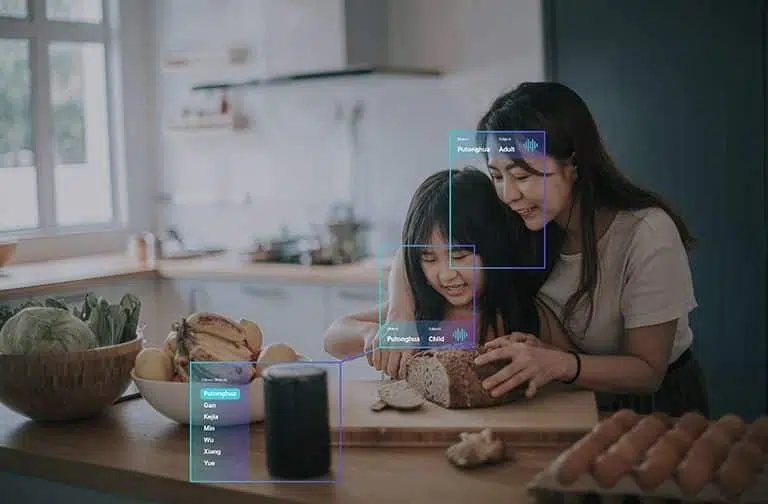
So that’s where it helped formed the company Shaip, which I lead right now as a CEO and co-founder. With Shaip, we took the first product in Amazon, to help them to launch Alexa into 25 different countries. Since we started our journey in medical transcription, we had a proprietary technology platform that we used for solving this particular use case, where we need to bring different speakers just like you and I talking, to different speakers in different languages, and capture the audio on different topics, and then do the transcription with 15-second segments. And if there is any background noise, we looked at the background noise that this is somebody opening the door, some TV in the background, or fan noise. So when the data is fed to the machine, it can ignore all those noises and also understand that what is the fan noise versus TV noise versus low noise, and then focus on the actual communication, actual speech data, and then align that with the 15-second segments transcribed to build different speech. So that’s how Shaip really started.
But I just wanted to note, again, that we are not just a healthcare AI company. Shaip is now helping many companies who have AI initiatives within their company, where they are solving any use case. They are generally categorized into text, audio, video, images, and 3D nowadays. So any of those audio, again, text, video, image, and 3D – whatever you need to scale, whatever data needed, with our expertise on launching our own AI company – we help our customers to pick the right data samples, right data sets, how to create the cohort, and work with them to define the guidelines before they hire us to work with them. Because if you don’t have the right code, standard data, a proper understanding of the use case, and proper guidelines to do the rotation, your AI model will not function the way you want to function. So that’s why we help our customers, and that’s where we are differentiating ourselves. We know what you are doing and what you want to do so that we can help you from the get go in capturing the right data sets and operating the right data sets with proper guidelines, and then give you that output point so you can train your models. So again, that’s what the Shaip platform and Shaip is about.
Medgadget: These days, when we say “AI” or “machine learning,” a lot of people may not actually know what that entails, and that it could be as simple as “a + b + c.” Can you describe to those who aren’t as familiar with ML and AI: what are the actual building blocks – in terms of Lego blocks – for constructing an AI model in healthcare?
Mr. Ghiya: So as you mentioned, AI has been used very loosely many times. And it could be as simple as two plus two is, or it could be as simple as detecting diabetes just by looking at your retina. That’s the technology that Google has done. So, for any use case, the journey starts with gold standard data. So no matter what field, whether it’s healthcare, autonomous vehicles, agriculture – any use case you want to solve – you first you need to define what AI problem we want to solve.
Let’s take the example of healthcare as we’re speaking about healthcare. So let’s say if you want to, in our case, read medical records and understand, like a physician or a medical coder, what is written in the document. You’d have to start from Ground Zero: imagine the medical coder going to the coding school on the very first day. And they don’t know anything about medical records, they don’t know anything about the course, and they don’t know anything about ICD-10, CPT code, so, you have to take the baby steps. First, we have to identify the appropriate cohort: this means the combination of notes or data that can represent a good cross section of various data points that can help the computer learn. And then you start with a simple application: that these are medical records, this is all the different titles you have that this is a history of present illness, this is a follow-up note, this is why, etc., so you start annotating all those basic things, and then you feed that to the machine so the machine can understand all the headers, all the medical records – these are the vitals, these are the medications. Then you take a different little bit of more complex notation, for example, if the documentation says take, let’s say, aspirin 2 times a day for next 10 days – so then it is by doing proper annotation with each machine that, okay, 2 times a day is a frequency, and 500 milligrams is a dosage, and 10 times 10 days is the course of the other medication. So, then you start doing the complex annotation and start building what we call ontology where all these medical concepts and everything is linked together to create a big knowledge base, basically.
And then you start going into more and more complex stuff, where even, you know, one report has some documentation of, let’s say, heart failure, complaint of, you know, congestive heart failure. And the lab reports the ejection fraction, so we can kind of correlate based on our knowledge base that these two are correlated. And if your ejection fraction is less than 40%, then you have a serious risk of attack, and you need to have the defib in this case implanted, and so on. That intelligence you have to kind of annotate, and then use computers to do the machine learning. So that’s how the healthcare reality can at least start in from the text documents. Now, if you want to solve the image API, or the video API, you need the data of various images or various videos of whatever use case you want to solve and do the annotations.
Medgadget: Can you give an example for solving video APIs and for 3D applications?
Mr. Ghiya: Okay, so 3D LIDAR is becoming very popular, especially in the autonomous vehicle sector. Or the lights of a Tesla. I’ve been in talking to many companies meetings who want to get into this race of EVs (electric vehicles) and autonomous vehicles. So they put the vehicle on the ground, and they take 3D videos. And instead of doing the 2D annotation, now they’re looking for three dimensional annotations so they can identify the objects, other vehicles, or whatever it is more precisely so as they’re rolling out these autonomous vehicles, they can be safer and better, basically. So that’s what happens in the 3D space. In the healthcare industry, they some of very sophisticated machines nowadays that take the images in 3D. So you need the proper platform and proper tools to take that input. And then, let’s say, there are some machines now we can use to see 3D brain aneurysms. We can run that whole video on our platform and show the progress of the algorithm how it’s happening in the brain by doing the simulation. Those things are coming more and more.
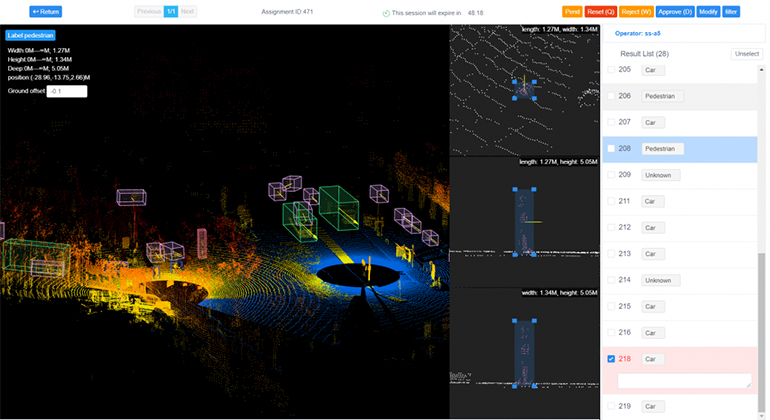
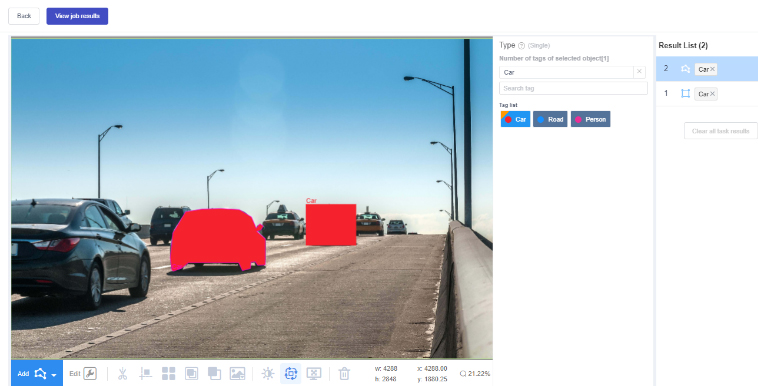
To speak on video – a lot of applications are coming into the drone videos. So we are working on few of them, let’s say one of them is drone videos flying over agricultural lands, and then capturing the video of the very large farms or a very large forest. And then let’s say they’re doing some AI on the crop, then we need to annotate. They take these drone videos at a regular intervals: either daily, weekly, or monthly, depending upon what use case they are solving. And then we have to annotate the progress of the crop, such as how it’s growing. So based on that, they can predict what is the right time to do the fertilization, what is the right time to intervene and do some chemical spraying, or what is the right time to actually raise the crop. And so those kind of use cases are happening with video. Then the other project we are doing – we have a company that wants to build a civilian system. So they are asking us to deploy the people to take the drone videos off the public places, government buildings, private factories, you know, private areas, from different heights, anywhere from 10 feet to 200 feet to 500 feet, and then, you know, run these sequence every day for 30 minutes, every hour, every two hours. And then do the video and rotation of the people moving so they can basically see the pattern of the traffic and try to predict if there is activity or any other activities, which are not normally going to happen or not. A lot of use cases are happening in the video spaces and it’s the same thing in healthcare.
Medgadget: So with all those use cases, how do you make sure you’re capturing the right context, especially in the 3D spaces?
Mr. Ghiya: So that’s very important. That’s what we call gold data, right? Gold Standard data, because the reality is going to be built on the data that you actually use at the beginning. So it’s very important that you capture that idea. If it is a very complex 3D use case, it’s still very common that the company themselves would create that data, because there is not a very common space right now and they don’t trust third party companies to create this content and will have their own content instead. Then they will either tell us that they use the same guidelines and replicate the same kind of videos or reverse that data. And then they don’t have a platform, they don’t have the labor pool. And if they do, it’s very expensive, because what is happening nowadays is that data scientists are many times becoming data editors. And what I mean by that is, you know, data scientists get paid at a very high level, based on their salary level a data scientists, but they end up spending a lot of time in cleaning the data and analyzing the data, which can be done at a very low cost, if you’re the right platform, and if you’re using the right tool, and if somebody understands that.
So in some cases, the data is provided by the companies. And if we ever capture it, then we bring in our subject matter experts, and we have a detailed conversation for hours, to understand what use case they are solving. And then once they have it, then, another very large tech company – one of the largest, I cannot disclose their name because of the agreements – want us to capture some videos using their devices. So we work with them in the beginning to really understand exactly what they want to solve, what kind of global footprint they want, in what country they want to capture this data, what age group, what gender, whether they want disabled people or regular people. So we have a discussion for days, going back and forth, before we sign off on these projects, and then once the proper understanding and sign off happens, that’s when we require people to capture these videos. And then first, what we do is we, let’s say product is, you know, to keep it simple: 100 videos. We take five videos based on all these guidelines, and give it to the company, so they can validate it, and they can then tell us that, okay, out of five, three, were perfect for these two, we need to do this and whatnot. So we call that a mini database. So for the mini database, before we kind of, you know, ramp up for the entire project, we focus on those 5% of the mini database, make sure it’s capturing what the company is actually looking for. And it’s going to help them to solve the use case they’re solving. And then if the requirement changes, then we documented the question and answer. And then we go into the full-fledged production. So that’s the kind of approach that we take. In any product, it’s very slow in the beginning, then it ramps up, and with a lot of feedback, it really ramps up fast. That’s how we typically solve this problem.
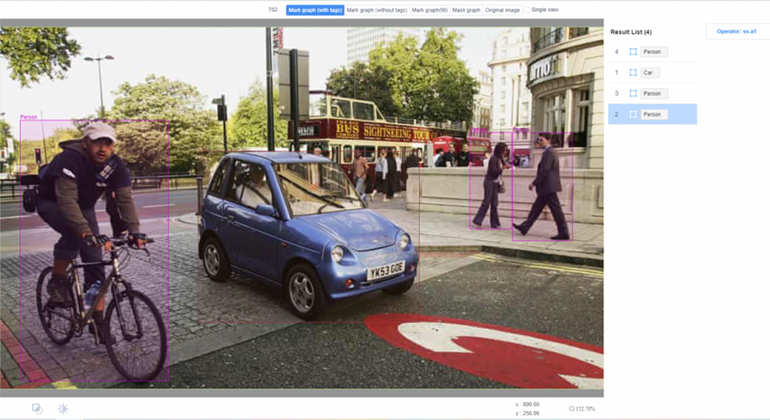
Medgagdet: Can you please talk more about maximizing the value of unstructured data – more specifically, how you create, license, or transform unstructured healthcare data into highly accurate and customized training data for machine learning algorithms? And what are the biases in that data?
Mr. Ghiya: So this is very important. Again, we need to understand the bias of the data, because in our own AI company, before I launched Shaip, we were competing against the likes of IBM’s Optim. And Optim is actually known to have a very huge bias in their AI output there. And it’s publicly available data. So, you know, what every you want to put in to develop these models, your model is going to predict based on what you are inputting, so it’s very important that you select the right data.
So, if you’re solving healthcare AI use cases for cancer, and then if you select all the “white males” in a certain age group, then you are introducing bias to your models. So that’s where you have to be very careful to select the data where you have, you know, proper ratios of different ethnicity, different age groups, and then based on that, you build your AI models. So, you know, again, in Optim’s case, their AI was biased towards white males and females, because that’s how they have captured that initial cohort of the data sets. And they kept on building based on that. And again, this is not to bad mouth any companies – the point I’m trying to make is to avoid data bias, it’s very important that you do heavy lifting in the beginning and understand the use case and understand that you don’t introduce any bias into your AI models by capturing the right data cohort.
And that kind of creating is done in very minute detail. So, just to give you an example, we help large companies in healthcare do speech-to-text models (audio AI) – and to avoid bias, we have to make sure that we have a proper ratio of male and female physicians, and proper ratios must have different, again, ethnicities and different specialties. You cannot introduce all the data from primary physicians or urgent care physicians – you have to make sure you have all the specialties covered equally. And based on how it goes, it sounds simple, but it goes back to what you said you’re solving and then do the reverse math and then understand what are the parameters which are going to influence the output to solve this use case, and then make sure that you have enough variety of the data and the cohort, so that you’re not introducing any bias to the model.
Medgadget: What are some of the challenges you faced with legislation and limiting such as GDPR and HIPAA?
Mr. Ghiya: GDPR and HIPAA both have the requirements. They want to make sure that all the PHI and PII is properly redacted. And before any of the data goes out to train any of the models. So for the right reasons, all these government agencies are in existence. And what Shaip has done is, you know, we have most of the GDPR and HIPAA compliance certificates from a third party who comes and goes through the process of how we are removing the PII and PHI from the healthcare records, or the financial documents or whatever we have, and what are the processes and how we are documenting it, and they look at the output that we have. And then they give us the certification. So in our opinion, there are a lot of companies coming up in this space. But it is very important for everybody to understand HIPAA and GDPR compliances and then comply with them because you are dealing with lot of sensitive data here. And when we are working on the healthcare AI, or even any image or video, a lot of public data is coming to our various sources.
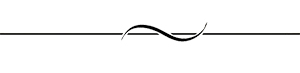
Link: Shaip company homepage…